Case overview
In a churn prediction project, the focus lies on forecasting customer attrition, a critical metric for businesses. By leveraging data analysis and predictive modeling techniques, the project aims to anticipate the percentage of customers who may discontinue using the company’s products or services. Recognizing that acquiring new customers often incurs higher costs compared to retaining existing ones, the project seeks to empower the business with insights to proactively address potential churn and foster customer retention strategies.
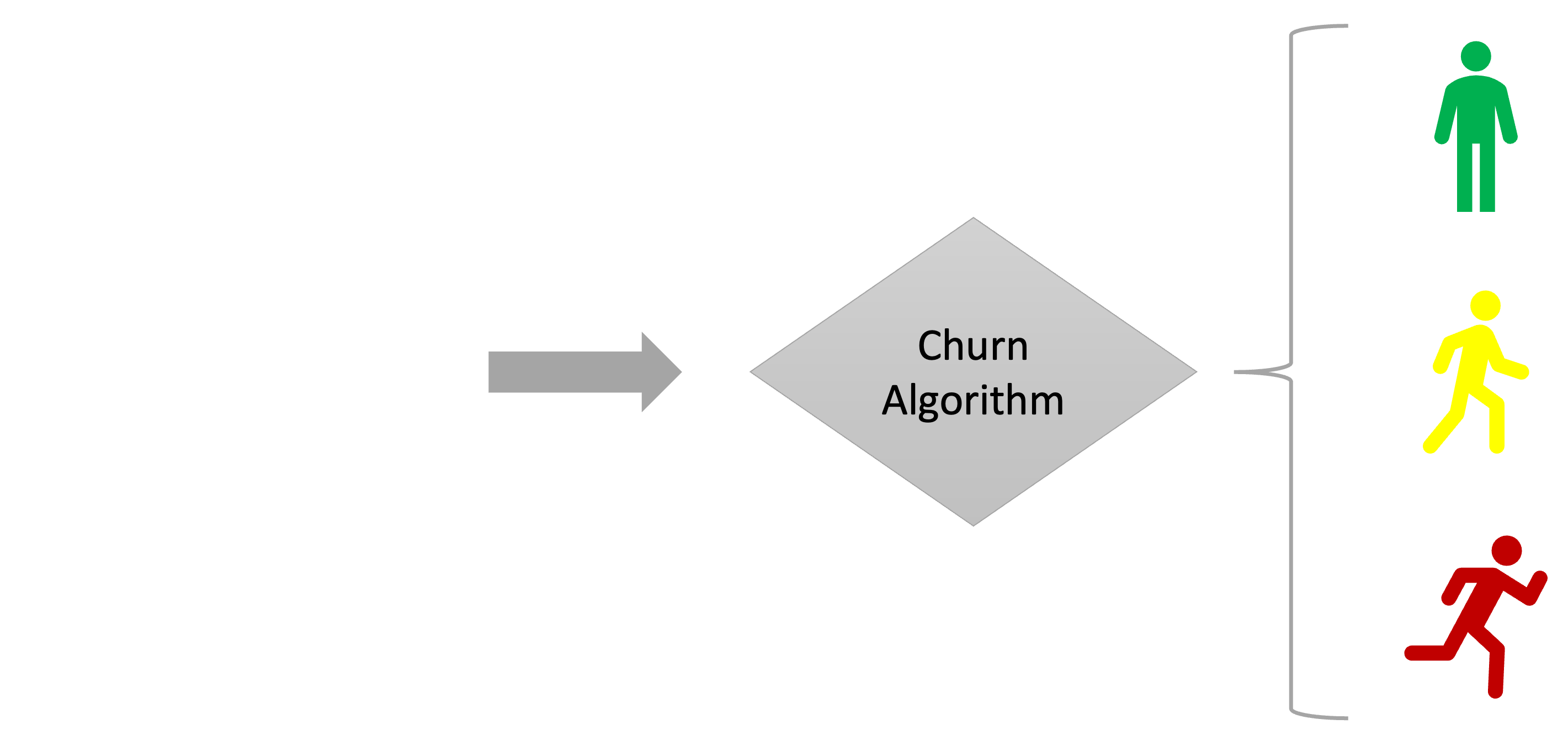
Churn definition
Defining what we mean by churner is mandatory: any further analysis will have a comparative nature, thus we have to identify a criteria to split the customers base between churners and non churners.
Some of the key points:
- Context matters
- Timeframe
- Impact metric
Features identification
Identifying the right features is crucial for building a successful model that can accurately predict which customers are likely to leave. This process involves understanding the different types of features available, selecting the most relevant ones, and preparing them for model training.
Model training
Several machine learning models have been investigate and trained: each approach has strengths, weaknesses.
- Logistic Regression
- Decision Trees
- Support Vector Machines
- Neural Networks
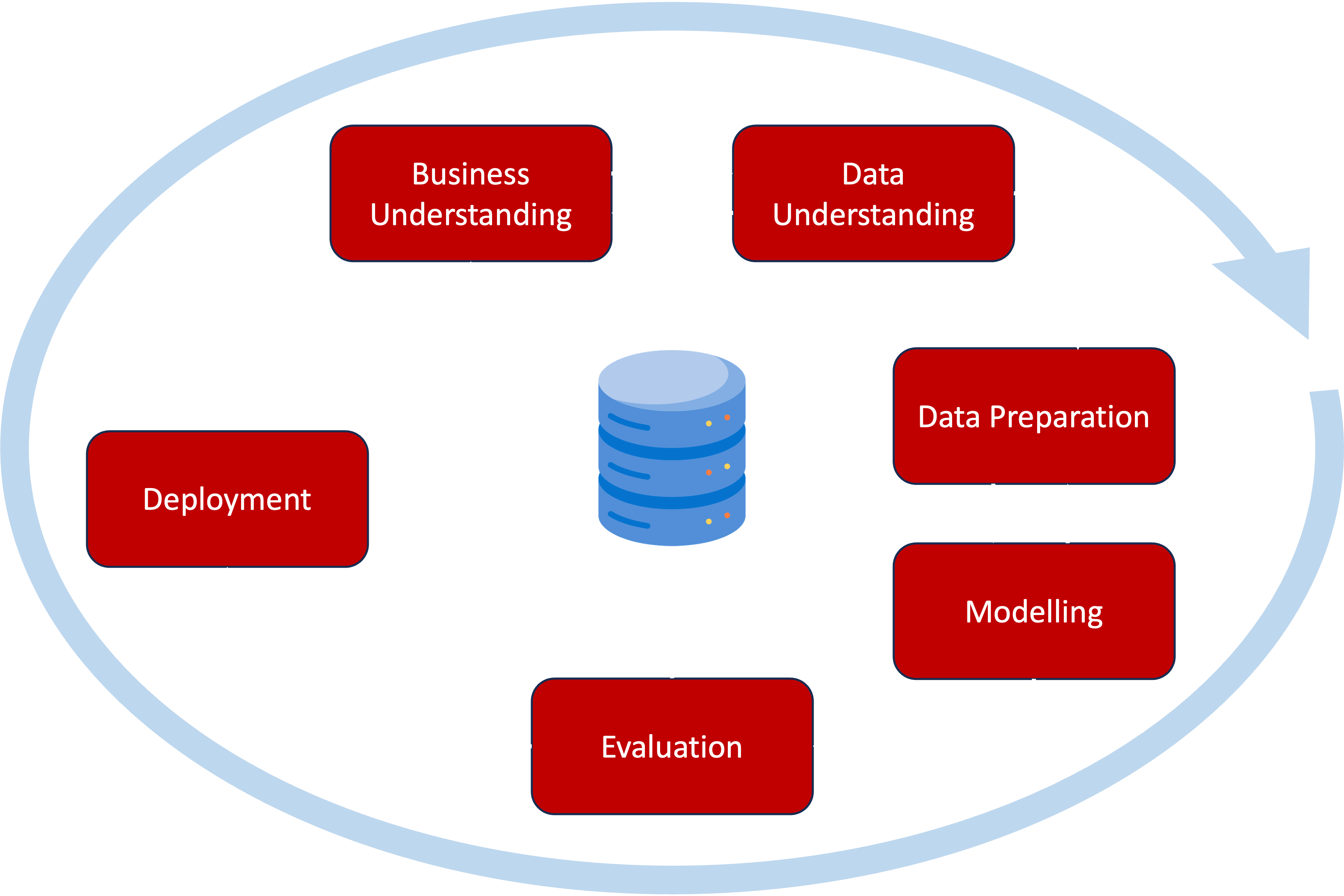
The Solution
We explored various approach and developed a final model to predict customer churn with the analogy of “customer temperature” ranging from hot (highly engaged) to frozen (likely to churn).
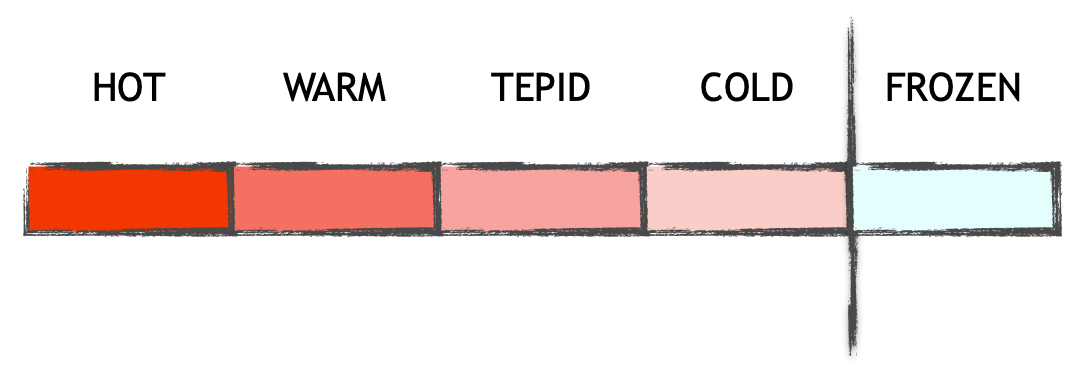
Results
By conceptualizing customer churn in terms of “temperature,” businesses prioritized their retention efforts more effectively. For instance, company allocated resources to delight hot customers, implement targeted interventions to re-engage warm and cool customers, and develop win-back strategies for cold customers. Additionally, by leveraging data-driven models to predict customer temperature and churn propensity, businesses proactively identified at-risk customers and intervene before they churn, ultimately maximizing customer retention and lifetime value.